|
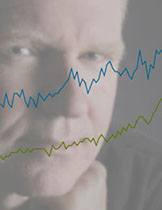
|
Thanks for taking the time to read my thoughts about Visual Business
Intelligence. This blog provides me (and others on occasion) with a venue for ideas and opinions
that are either too urgent to wait for a full-blown article or too
limited in length, scope, or development to require the larger venue.
For a selection of articles, white papers, and books, please visit
my library.
|
|
April 18th, 2017
This blog entry was written by Nick Desbarats of Perceptual Edge.
Should you include grid lines in your graph? If so, how many? Is it O.K. to add another variable to your graph by encoding it using size (for example, by varying the size of points in a scatterplot) or color intensity (for example, by representing lower values as a lighter shade of blue and higher ones as darker)? How small can you make your graph before it becomes problematic from a perceptual perspective? These and many other important graph design decisions depend, in part, on the degree of precision that we think that our audience will require. In other words, they depend on how precisely we think that our audience will need to be able to “eyeball” (i.e., visually estimate) the numerical values of bars, lines, points, etc. in our graph. If we think that our audience will require no more than approximate precision for our graph to be useful to them, then we can probably safely do away with the gridlines and add that other variable as color intensity or size. If we have limited space in which to include our graph on a page or screen, we could safely make it quite small since we know that, in this particular case, the reduction in precision that occurs when reducing a graph’s size wouldn’t be a problem.

Our audience won’t be able to eyeball values all that precisely (what exactly are Gross Sales or Profit for the South region?), though such a design would provide enough precision for our audience to see that, for example, the West had the highest Gross Sales, and that it was about four times greater than the East, which had relatively low Profit, etc. Despite its lack of high precision, this graph contains many useful insights and may be sufficient for our audience’s needs.
If, on the other hand, we’ve spoken to members of our audience and have realized that they’ll need to visually estimate values in the graph much more precisely in order for the graph to be useful to them, then we’re going to have to make some design changes to increase the precision of this graph. We might need to add gridlines, break the quantitative scale into smaller intervals, find a more precise way to represent Profit (for example, by encoding it using the varying lengths of bars or the 2D positions of points), and perhaps make our graph larger on the screen or page.

As you probably noticed, adding gridlines, making the graph larger, and breaking the quantitative scale into smaller intervals all came at a cost. While these changes did increase the precision of our graph, they also made it busier and bigger. Being forced to make these types of design trade-offs is, of course, very common. In nearly every graph that we design, we must struggle to balance the goal of providing the required level of precision with other, competing goals such as producing a clean, compact, uncluttered, and quick-to-read design.
What if we know, however, that our graph will only ever be viewed in a software application that supports tooltips, i.e., that allows our audience to hover their mouse cursor or finger over any bar, line, point, etc. to see its exact textual value(s) in a small popup box?

In this case, perfect precision is always available if the audience ever needs to know the exact value of any bar, point, etc. via what researcher Ben Shneiderman termed a “details-on-demand” feature. In the 1990’s, Shneiderman noted that suppressing details from a visualization and showing specific details only when the user requests them enables the user to see a potentially large amount of information without being overwhelmed by an overly detailed display. A well-designed visualization enables users to see where interesting details may lie and the details-on-demand feature then enables them to see those details when they’re needed, but then quickly hide them again so that they can return to an uncluttered view and look for other potentially interesting details.
So, does the availability of details-on-demand tooltips mean that we can stop worrying about precision when making design decisions and optimize solely for other considerations such as cleanness? Can we set the “precision vs. other design considerations” trade-off aside in this case? I think that the answer to this question is a conditional “yes.” We can worry less about precision if we know all of the following:
- Our graph will only be viewed in a software application that supports tooltips (which most data visualization products now support and enable by default). If we think that there’s anything more than a small risk that our audience will, for example, share the graph with others by taking a screenshot of it or printing it (thereby disabling the tooltips), then precision must become one of our primary design considerations again.
- Our audience is aware of the tooltips feature.
- Our audience will only need to know precise values of the bars, points, lines, etc. in our graph occasionally. If we think that our audience will frequently need to know the precise values, giving them a lower-precision graph with tooltips will force them to hover over elements too often, which would obviously be far from ideal. In my experience, however, it’s rare that audiences really do need to know the precise values of elements in a graph very often—although they may claim that they do.
If we don’t know if all three of these conditions will be true for a given graph, we don’t necessarily have to ramp up its size, add gridlines, etc. in order to increase its precision, though. If we have a little more screen or page real estate with which to work, another solution is to show a clean, compact, lower-precision version of our graph, but then add the textual values just below or to the side of it. If the audience requires a precise value for a given bar, point, etc. in our graph, it’s available just below or beside the graph.


If we think that, for example, our audience is going to be embedding this graph into a PDF for a management meeting (thus disabling the tooltips) and that higher precision will be required by the meeting attendees, this would be a reasonable solution. For some graphs, however, the set of textual values may end up being bigger than a higher-precision of version of the graph, in which case the higher-precision graph may actually be more compact.
As with so many other data visualization design decisions, knowing how to balance precision versus other design considerations requires knowing your audience, what they’re going to be using your visualizations for, and—particularly in this case—what devices, applications or media they’ll be using to view the visualization.
Nick Desbarats
April 10th, 2017
Despite the popular notion that we live in the Information Age, most organizations still base most of their decisions on gut instinct rather than information (i.e., evidence of what’s actually happening and what actually works). Intuition, based on true, hard-won expertise, has a role in organizational decision making, but many decisions, especially the most important decisions, should be rooted in evidence. In their excellent book, Hard Facts, Dangerous Half-Truths and Total Nonsense, Jeffrey Pfeffer and Robert I. Sutton wrote:
Business decisions, as many of our colleagues in business and your own experience can attest, are frequently based on hope or fear, what others seem to be doing, what senior leaders have done and believe has worked in the past, and their clearly held ideologies—in short, on lots of things other than facts…If doctors practiced medicine the way many companies practice management, there would be far more sick and dead patients, and many more doctors would be in jail.
We cannot know how well we’re performing without evidence of what’s going on and what works. To improve performance, we must measure it. Relatively few organizations can prove that they’re performing well, but they certainly spin great yarns trying to convince us and even themselves. According to Stacey Barr, high-performance organizations can prove their success with facts. In her efforts to help organizations enter the realm of high performance, she has written a new book to make the case for evidence-based leadership titled Prove It! How to Create a High-Performance Culture and Measurable Success.
Here is the opening paragraph of Chapter 1:
Almost any organisation can prove that it does things. It can prove that it hires people, that those people carry out different tasks, and that money is earned and spent. But what many organisations cannot prove is the most important thing: whether they are fulfilling their purpose or not. High-performance organisations don’t just do stuff. They have an impact—ideally, the impact they exist to make. And they can prove how much impact they create.
She doesn’t just make the case for evidence-based leadership, but also explains in concise, clear, and readable prose how to achieve it. This book is short, sweet, and practical. It is also incredibly smart. If you are an information worker, but are frustrated because you work in an organization that doesn’t base its decisions on evidence, despite what it claims, this is the book that you should place in the hands of your organization’s leaders. Let Stacey Barr make the case for organizational improvement through better evidence-based leadership for you. Without buy-in from your executives, you don’t have a chance.
Take care,

April 5th, 2017
You might consider the way a humble and common line graph works to be obvious. If you learned to use them in the workplace, chances are that you think of line graphs as a conventional means to display one or more sets of time-series values. This is the graphical use of lines that typically bears the name “line graph.” Confusion is created, however, by the fact that lines can be used for various purposes in graphs. One common use is lines or curves of best fit, also known as trend lines or fit models. These lines summarize patterns in quantitative data sets rather than representing the actual values on which that summary is based. Lines are also used to represent multivariate data in the form of parallel coordinates plots, which work quite differently from regular line graphs. There is another graphical use of lines that you have been exposed to if you’ve studied mathematics beyond a basic level: equation graphs and function graphs. These graphs do not represent actual values that have been collected, but instead model mathematical equations and functions, representing visually what can also be expressed using mathematical notation. If you’ve been exposed extensively to this graphical use of lines, you might find regular lines graphs a bit confusing and misleading, despite their simplicity.
Every once in a while when teaching my Show Me the Numbers course about table and graph selection and design, a participant expresses concern that my use of line graphs is misleading. I’ll illustrate this situation with an example. The graph below is a simple, typically designed line graph that displays one set of time-series values.

This line graph displays only 10 quantitative values. The values are positioned as points along the line, one for each year. The line connects the 10 data points as straight segments from one data point to the next. For this reason, what I’m calling a typical line graph is sometimes referred to more specifically as a segmented line graph. The purpose of the line is to clearly display the pattern of change along the time series. The quantitative values are aggregates—in this case sums of profits—for each interval of time along the X axis. The slope of each line segment represents the nature of the change between each contiguous set of intervals (e.g., between 2009 and 2010). Nowhere between contiguous data points do any other values exist. Stated differently, no values can be read along a line apart from a single data point for each interval.
The objection that has been expressed in my classes a handful of times usually goes something like this: “That line graph is misleading. It claims that from 2009 to 2010 the profits decreased by a constant amount during that period of time, but there was probably a great deal of variation in daily profits.” This objection is usually based on a misunderstanding that stems from experience using lines to graph mathematical equations and functions. When a line is used for that purpose, we don’t usually call it a line graph. Instead, it is a “graph of an equation” or a “graph of a function,” among other potential terms. With graphs of equations or functions, every possible position along the line represents two values, one associated with the X axis and another associated with the Y axis. The scales along both axes are continuous quantitative scales. Given this mathematical use of lines in graphs, we can certainly understand why someone might find the way that a regular line graphs work for displaying time series to be confusing, resulting in the concern that they sometimes express. If you are one of those who shares this concern, just realize that lines are used for various purposes in graphs and that, in regular line graphs for displaying time series, they work differently than for mathematical equations and functions.
In a regular line graph of a time series, the scale along the X axis is what’s called an interval scale. It functions as a type of categorical scale that labels what the values represent. An interval scale begins as a range of quantitative values, which is then subdivided into a set of equally sized intervals, and a label is associated with each to identify it (e.g., >=20 & <30, >=30 & <40, etc.). Time is quantitative in nature. By this I mean that you can sum the number of hours, days, weeks, months, etc., to quantify the duration of time that has transpired between any two points in time. When we subdivide time into intervals of equal size, we express time as a categorical scale of an interval type. (Even though months are not all equal in size, when we display a times series by month, we treat it as if the months are equal when they are close enough in size to suit our purpose.) The intervals are not specific points in time, but are instead specific ranges of time. In a line graph that displays a single set of time-series values, one and only one value appears for each interval, which usually consists of an aggregate value per interval (most often a sum, and secondarily an average), but on occasions, rather than aggregates, the value for each interval is a measurement taken at a particular point in time (e.g., the closing price of a stock with daily intervals).
A great deal of confusion results from the many ways that we graphically represent data and the sloppy terms that we use to describe them. We would all benefit from a better understanding of the fundamentals and more clearly defined terms.
Take care,

March 3rd, 2017
Even though I’ve questioned the usefulness and integrity of Gartner’s Magic Quadrant many times when it’s been applied to products related to analytics and data visualization, I’ve recently realized that there’s at least one aspect of the Magic Quadrant for which we should be grateful: the honesty of its name. By calling the quadrant “magic,” Gartner helpfully hints that it should not be taken seriously—it’s magical. We should approach it as we would the performance of a stage magician. When reading it, we should suspend disbelief and simply enjoy the ruse. Gartner’s Magic Quadrant is an act of misdirection, sleight of hand, smoke and mirrors. Understood as such, it’s grand entertainment.
Gartner recently published the 2017 edition of its “Magic Quadrant for Business Intelligence and Analytics Platforms.” As in past years, it is not a valid assessment of the products and vendors. Unfortunately, however, it will nevertheless be used by many organizations to select products and vendors. There is a dirty little secret about the Magic Quadrant that most industry leaders won’t publicly admit: few of them, including the vendors themselves, take the Magic Quadrant seriously as a valid assessment. They laugh about it in whispers and behind closed doors. They do take it seriously, however, as a report that exercises an undeserved degree of influence. The Magic Quadrant is a highly subjective evaluation of products and vendors that reflects the interests of the vendors that appear on it and Gartner’s interests as well, for Gartner is paid dearly by those vendors. Gartner’s coverage is about as “fair and balanced” as Fox News.
Although it should always have been included in any serious review of Business Intelligence tools, it took Gartner several years to shift the focus of its Magic Quadrant for Business Intelligence to one that supposedly embraces the importance of analytics (i.e., data sensemaking)—a transition that Gartner now claims is complete. Unfortunately, Gartner does not understand analytics very well, so it bases its evaluation on criteria that reflect the interests and activities of the vendors rather than a clear understanding of analytical work and its requirements. The criteria largely focus on technical features rather than on the fundamental needs of data analysts, and many of the features on which it bases its assessment are distractions at best, and, in some cases, recipes for disaster.
I won’t take the time to critique this year’s Magic Quadrant in detail, but will instead highlight a few of its flaws.
The Magic Quadrant displays its findings in a scatterplot that has been divided into four equal regions: “Niche Players,” “Challengers,” “Visionaries,” and “Leaders.” As with all scatterplots, a quantitative scale is associated with each of the axes: “Completeness of Vision” on the X-axis and “Ability to Execute” on the Y-axis.

The actual measures that have been assigned to each vendor for these two variables are not shown, however, nor are the underlying measures that were combined to come up with these high-level measures. Gartner is not willing to share this data, so we have no way to assess the merits of the results that appear in the Magic Quadrant. Even if we could see the data, the Magic Quadrant would be of little use, though, for it doesn’t measure the most important qualities of BI and analytics products, nor is it based on data that is capable of assessing the merits of these products. We cannot actually measure a vendor’s ability to execute or its completeness of vision. Gartner’s conclusion that the vendors with the most complete visions are Microsoft, followed at some distance by Salesforce and the ClearStory Data, is laughable. The visions that place these vendors at the forefront in the Magic Quadrant will not lead to improved data sensemaking. Something is definitely wrong with the way that vision is being measured.
If I decided to use a scatterplot to provide a summary assessment of these products, I would probably associate “Usefulness” with one axis and “Effectiveness” with the other. What matters most is that the tools that we use for data sensemaking provide the functionality that is most useful and do so in a way that works well.
The Magic Quadrant is almost entirely based on responses to questionnaires that are completed by the vendors themselves and by those who use their products. This is not the basis for a meaningful evaluation. It is roughly equal to evaluating Mr. Trump’s performance by asking only for his opinion and that of those who voted for him. The degree of bias that is built into this approach is enormous. Obviously, we cannot trust what vendors say about themselves, nor can we trust the opinions of those who use their products, for they will almost always be biased in favor of the tools that they selected and use and will lack direct knowledge of other tools. The best way to evaluate these products would involve a small team of experts using a good, consistent set of criteria to review and test each product as objectively as possible. Questionnaires completed by those who routinely use the products could be used only to alert the experts to particular flaws and merits that might not be obvious without extensive use. Why doesn’t Gartner evaluate the field of vendors and products in this manner? Because it would involve a great deal more work and require a team of people with deep expertise acquired through many years of doing the actual work of data sensemaking.
Immediately following a two-sentence “Summary” at the beginning of the report, Gartner lists its “Strategic Planning Assumptions,” which are in fact a set of six prognostications for the near future. Calling them assumptions lends credence that these guesses don’t deserve. They are not predictions based on solid evidence, but seem like more of a wish list of sorts. Let’s take a look at the list.
By 2020, smart, governed, Hadoop/Spark-, search- and visual based data discover capabilities will converge into a single set of next-generation data discovery capabilities as components of modern BI and analytics platforms.
At least one member of Gartner’s team of BI analyst must have a marketing background. This is meaningless drivel that can neither be confirmed nor denied in 2020.
By 2021, the number of users of modern BI and analytics platforms that are differentiated by smart data discovery capabilities will grow at twice the rate of those that are not, and will deliver twice the business value.
For some unknown reason, this prediction will take a year longer than the others to be realized. What are these “smart data discovery capabilities?”
Smart data discovery — introduced by IBM Watson Analytics and BeyondCore (acquired by Salesforce as of September 2016) — leverages machine learning to automate the analytics workflow (from preparing and exploring data to sharing insights and explaining findings). Natural-language processing (NLP), natural-language query (NLQ) and natural-language generation (NLG) for text- and voice-based interaction and narration of the most statistically important findings in the user context are key capabilities of smart data discovery.
First off, I hope this doesn’t come true because these so-called “smart data discovery capabilities” are almost entirely hokum. Relinquishing control of data sensemaking to algorithms will be the death of meaningful and useful analytics. Regardless, there is no actual way to confirm if those who use these capabilities will “grow at twice the rate” of those who don’t, and there certainly isn’t a way to measure a two-fold increase in business value. Even if they defined what they mean by these measures, they wouldn’t have a way to gather the data.
By 2020, natural-language generation and artificial intelligence will be a standard feature of 90% of modern BI platforms.
This is somewhat redundant because Gartner defines smart data discovery, addressed in the previous prediction, as products that incorporate machine learning and natural language processing. I’m assuming that by “artificial intelligence” Gartner is actually referring to machine learning algorithms, because none of these products will incorporate true AI by 2020, and probably never will.
By 2020, 50% of analytic queries will be generated using search, natural-language processing or voice, or will be autogenerated.
According to this, by 2020 the 90% of BI products that incorporate natural language processing will be used to generate 50% of all queries through natural language interfaces. That sounds cool, but isn’t. Natural language is not an efficient way to generate data sensemaking queries. Anyone who knows what they’re doing will prefer to use well-designed interfaces that allow them to directly manipulate information and objects on the screen rather than using words.
By 2020, organizations that offer users access to a curated catalog of internal and external data will realize twice the business value from analytics investments than those that do not.
What do they mean by a “curated catalog?” Here’s the closest that they come to a definition:
A curated agile data catalog where business users can search, access, find and rate certified internal data as well as open and premium external data with workflow — in order to promote harmonized data to certified status — is becoming key to governed modern deployments leveraging complex distributed data with an increasing number of distributed content authors.
This is mostly gobbledygook. Without a clear idea of what this is, this prediction can never be confirmed, and even if the meaning were clear, we would not be able to determine if these features led to “twice the business value.”
The sixth and final prediction is one of my favorites:
Through 2020, the number of citizen data scientists will grow five times faster than the number of data scientists.
As I’ve written before, there is no science of data. The term data scientist is a misnomer. Even if this were not the case, there is no commonly accepted definition of the term, so this prediction is meaningless. Now, add to this a new term that is even more meaningless—“citizen data scientist”—and we have the makings of complete nonsense. And finally, if in 2020 you can demonstrate that so-called citizen data scientists grew five times faster than the number of so-called data scientists, I’ll give you my house.
It’s ironic that Gartner makes such unintelligent statements about business intelligence and such unanalytical statements about analytics and then expects us to trust them. Unfortunately, this irony is missed by most of the folks who rely on Gartner’s advice.
Take care,

February 6th, 2017
I began talking about finding and then telling the stories that reside in data 13 years ago, several years before “data storytelling” became a common expression and popular pursuit. Mostly, I was speaking of stories metaphorically. In some respects, I regret using this expression because, like many metaphors, its use has become overblown and misleading. I did not mean to suggest that stories literally reside in data, or, if I did, I was mistaken. Rather, facts reside in data from which stories can sometimes be woven. Literally speaking, storytelling involves a narrative presentation that consists of a beginning, middle, and end, along with characters, plots, and often dramatic tension. Data does not tell stories, people do.
Don’t be misled: data storytelling (i.e., the presentation of data in narrative form) makes up a tiny fraction of data visualization. The vast majority of data visualizations that we create present facts without weaving them into stories. Relatively few of the facts that we display in data visualizations lend themselves to storytelling. I’m not diminishing the usefulness of data storytelling, which can be incredibly powerful when appropriate and done well. I’m merely pointing out that data storytelling is not some new endeavor or skillset that dominates data visualization. It is a minor—but nonetheless important and useful—aspect of data visualization. Not everyone who works in the field of data visualization must be a skilled storyteller. In general, it’s more valuable to be skilled in data sensemaking and graphicacy, as well as a clear thinker and communicator, and to possess knowledge of the data domain.
When facts can indeed be woven into a story, however, do so if you know how. We love stories. They can breathe life into data. Just don’t try to impose a story on a set of facts to create life where it doesn’t exist.
Take care,

|